October 27, 2023
The future of Generative AI language models is “small”
By Liza Colburn
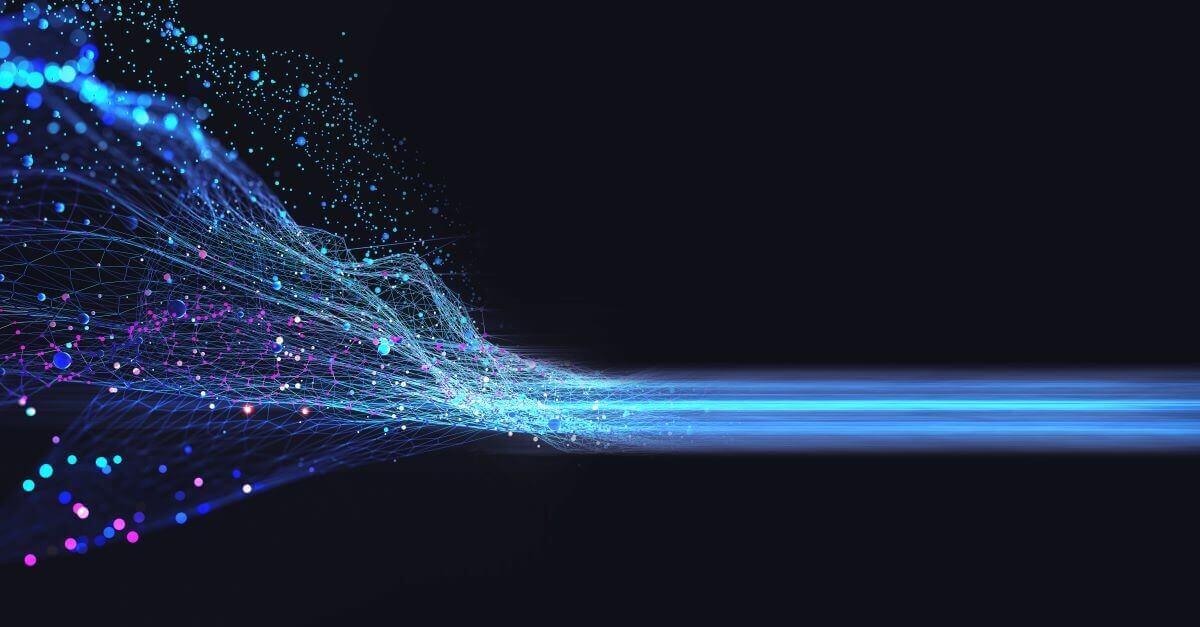
The furor has calmed since last November when Generative AI first rose to peak hype. The breathless excitement about the size of OpenAI’s GPT-4, Google’s Bard, and Meta’s LLaMA and the broad range of tasks they can fulfill has given way to more measured consideration of the value they bring to enterprise buyers. Are the number of GenAI training parameters relevant for an enterprise with a specific business goal? Might a smaller model trained only on relevant language data be the future of Generative AI?
This is an age-old question in the tech space. Many technologies enter mainstream awareness with a general-purpose application that must evolve to serve enterprise needs. Take speech recognition as an example. Applying the technology to drive customer service prompts required a different model from applying the technology to identify a customer for security purposes, or disambiguating the street names “Market” from “Marquette” for navigation guidance.
A similar evolution is already underway with Generative AI. Commentators have been arguing that LLMs will get smaller, faster, and less expensive to build and use as enterprises identify specific use cases and develop specific models for them. We share our own perspective specifically for marketing use cases in our CMO’s Guide to Achieving Impact With Generative AI.
In this post, I discuss why smaller may be better for enterprise technology buyers looking to understand the future of Generative AI.
What are the large Generative AI language models good for and why?
LLMs like GPT-4, Bard, and LLaMa represent a massive step forward in the discipline of natural language processing (NLP). Trained on a vast corpus of general-purpose text from the internet, they work by predicting the next likely word or set of words in a sentence, based on what they’ve seen in their training data.
When they first achieved mainstream attention, there seemed to be endless potential for them to evolve toward artificial general intelligence, a theoretical model for an AI that can reason through any task.
The current reality looks a bit different. Based on the nature of their training data and how people are using them, there appear to be three immediate use cases for Generative AI.
Primary short-term use cases for LLMs
The first and most straightforward GenAI use case focuses on tactical writing tasks. No surprise there!
Depending on the user’s purpose, that text might be used as a first draft for some written output, such as an email, a resume, or a report. Gartner predicts that by 2025, 30% of outbound marketing messages from large organizations will be synthetically generated, up from less than 2% in 2022.
The second immediate use case is to replace search with a more fluent and specific alternative. Over the past year or so, millions of people have used ChatGPT to answer a question that in the past they would have posed to a search engine. Instead of search results, snippets, or FAQs responses, they get full sentences. They can even get source materials if they ask.
Third, GenAI is emerging as a powerful tool for mining language data for ideas, opportunities, or sources of risk. For example, financial institutions and retailers are using it to mine transaction data to spot behavior associated with risk or fraud. Health researchers are hoping GenAI can help identify novel pharmaceuticals.
Beyond text, there are other use cases are emerging for the text-to-image models, which allow users to quickly translate a verbal request into a corresponding visual (i.e. “Create a photo-realistic painting of a rottweiler running after a ball on the beach”).
These use cases validate the importance of LLMs for solving broad and common problems related to completing basic writing or information-gathering tasks. They cannot do more than that on their own, however. To the extent that they do, it is because users are “hacking” the models with more sophisticated prompts.
What does that mean for enterprises?
What should an enterprise look for in Generative AI?
Employees write tactical texts and conduct both basic research and fact checks for their jobs all the time. For many of these general-purpose tasks, a core LLM large language model will do just fine.
Ask a general-purpose LLM to perform a specialized task for an enterprise, however, and that LLM will almost always underperform. It simply wasn’t trained to execute on specific language-driven tasks aligned to a concrete performance goal.
Look at that picture of a rottweiler as a low-stakes example. It is recognizably a dog in that breed. The fact that I could create it within less than a minute is a testament to the power of the underlying model. One reason why that speed is possible is because LLMs that are general in nature use a “limit approaches” construct to fulfill the purpose they were designed for. Put simply, they are designed to get close enough, not make an exact match. Fuzzy logic is both the power of a general-purpose LLM and its weakness.
Look again at that dog picture and fuzzy is what you get. For example, the foreleg and shoulder are clearly human with black fur on them.
Does it matter?
Not if all I am doing is using a picture to illustrate a point in a blog post. It would matter a great deal, however, if I was using a text-to-visuals model to design a product that has utility and safety requirements. Or reporting on public company sales data to the SEC. Or mapping precise exit routes for emergency evacuation procedures.
For important tasks aligned with business goals, enterprise buyers can’t tolerate imprecision. Yet that is inevitable when too many of a model’s training parameters are irrelevant to the task at hand. It is like asking a Boeing 747 to do the job of a General Dynamics F16. Both are aircraft, but the Boeing wouldn’t just be the wrong choice for an active combat zone. It would be a liability.
For that reason, the future of Generative AI for enterprises applications must meet standards in the following areas:
- Reliability—Does the model perform the task it says it will?
- Efficiency—Does the model perform the task faster and at greater scale than existing alternatives?
- Effectiveness—Does the model perform the task with higher quality or performance than existing alternatives?
- Security—Is the organization’s data and IP safe and protected?
- Innovation—Will the model solve a pressing business problem or enable new opportunities above and beyond what the enterprise could achieve without it?
How enterprises are using Generative AI models to solve concrete needs
The good news for enterprise technology buyers is that the options for enterprise Generative AI are already diverse and growing well beyond the familiar, consumer-oriented tools. The five standards of reliability, efficiency, effectiveness, security, and innovation are deeply embedded in the DNA of organizations creating Generative AI models and solutions from the outset to solve a defined business problem.
Nvidia, for example, has an enterprise Generative AI model it licenses for third-party developer adoption. So does Amazon for its AWS customer base. Large technology companies have also integrated Generative AI capabilities into their platforms. Examples include Microsoft 365, SAP, and Adobe Firefly. Finally, the start up community is bringing an array of specific point solutions with Generative AI models trained to solve specific problems.
5 functional capabilities enterprise-ready Generative AI must deliver
A key difference between enterprise Generative AI and general purpose LLMs lies in the following functional capabilities:
- Optimized user interface (UI) and user experience (UX). Enterprise solutions provide the user with a seamless and straightforward experience that allows them to optimize the benefits. When we think about interactions with the core models so far—ChatGPT as an example—the best experience comes to those who write well-crafted prompts that they create outside of the platform itself. An optimized UI/UX embeds optimized usage into the interface itself.
- Workflow integration. Enterprise solutions integrate into the organization’s processes and technology stacks, so that the user gains benefits in the context of how they already work.
- Metrics-based reporting. Enterprise solutions have built-in feedback loops, so that the user and the system itself gain insights into whether the outputs were “good”—meaning, whetherdid they meet the goal they intended to or not. The reporting capabilities also enable the system to learn and improve.
- Extension of the LLM capabilities. Enterprise solutions need a defensible extension and value-add beyond what a user could achieve with a general-purpose LLM. Those extensions also create a distinct value proposition that would be hard for a competitor to replicate.
4 GenAI options for enterprises
Given the use cases for Generative AI and the specific enterprise needs standards GenAI must address, consider the following four options for the future of Generative AI in your enterprise:
Option 1: Train your own
The launch of GPT-3 and others provided solutions to a few of the technical problems that made model development challenging for private enterprises. Specifically, the transformer architecture gave data scientists a new approach that sped up language model training. Any enterprise can theoretically use these techniques to create their own language models for in-house purposes, if they have the staff and the budget to do it.
Pros:
- You get a proprietary data model that rates high on security and innovation if trained with proprietary enterprise data.
- You may achieve efficiency in model performance (if not in model creation).
Cons:
- It’s still expensive and time consuming.
- It requires large, clean data usable for training purposes—which few organizations have due to privacy regulation, in-house policies, etc.
- You need dedicated resources to continue evolving the model according to a development roadmap—a difficult proposition if this is not your core business.
- You need to build in the user interface, workflows, and feedback loops into the model design.
Option 2: Leverage a general-purpose, pre-trained model and fine-tune it for a specialty purpose
Enterprises can license or download LLMs and refine their functionality. There are two primary methods for doing that. One is by fine-tuning the model using specialized data sets to narrow the parameters that the LLM uses. Another is through prompt engineering, which uses specific instructions to focus the LLM to deliver a specific output, sometimes by focusing only on certain parameters. Think of fine turning or prompt engineering as making a large language model intentionally smaller for a specific purpose.
Pros:
- You get improved reliability with both methods, with lower likelihood of hallucinations compared with un-tuned models.
- You may achieve efficiency in both model creation and performance by starting with an established working model.
- The established model may have a pre-built UI/UX
Cons:
- Effective fine-turning and prompting requires skilled data science talent.
- Not every enterprise has access to datasets they can use for fine-turning (due to privacy regulation, in-house policies, etc.).
- Putting enterprise data into a third-party model raises security concerns.
Option 3: Leverage Generative AI functionality embedded into enterprise software
As noted above, there have been a string of announcements from enterprise technology service providers about the Generative AI functionality they have developed for their solutions. Microsoft has integrated GPT-4 into Office 365; Salesforce has language generation capabilities in its marketing cloud, etc. The language model underpinning the Generative AI functionality may be large and general purpose, or it may be specialized, depending on how the vendor developed it.
Pros:
- You can efficiently access the Generative AI capabilities you already have in your tech stack and built into your workflows, often with no additional integration.
- High likelihood of reliable outputs.
- You may gain efficiency.
Cons:
- Use cases are limited.
- It locks you into one provider, or more likely a set of peripheral GenAI solutions. For example, marketing GenAI for email, text, or social posts that do not aggregate data or learn from each other, resulting in duplicate expense and fragmentation.
Option 4: Partner with point solution providers (like Persado) that have specialized GenAI capabilities for your use case
A range of point solutions have emerged to address specific Generative AI use cases. Persado Motivation AI, for example, is an enterprise Generative AI solution that optimizes the digital marketing language used to motivate customers to engage and act. Other marketing-focused solutions include Descript.ai for video editing and podcasting, and Peppertype.ai for content and video creation.
Some point solutions start with a licensed LLM and build their solution on top of it. Others, like Persado, build language models using specific data sets focused on the use case. In our case, we focus on brand campaign performance.
Pros:
- You can address specific problems with dedicated solutions for increased efficiency and (in some cases) effectiveness.
- The UI/UX, workflow integration, and data feedback loops are aligned with the specific purpose of the solution.
- There is a high likelihood of reliable outputs.
- Increased security.
Cons:
- Use cases are specific and limited to what the point solution is trained to do.
The future of Generative AI language models is smaller
The major Generative AI models brought the technology into the mainstream. For an enterprise to adopt a new technology widely, however, it must have a more precise business value proposition (efficiency, effectiveness, innovation) and clear governance parameters (reliability and security). While there will be a place for general purpose LLMs in that eco-system, there are also huge opportunities today for enterprises to adopt point solutions founded on smaller and more refined language models designed to solve a problem, or unlock an opportunity.