The CMO’s Guide to Achieving Impact With Generative AI
Generative AI has captured outsized attention from investors and enterprise leaders—so much so that
IBM expects to see a 4X increase in Gen-AI investment between 2023 and 2025. With marketing poised to be one of the most active early adopters, CMOs are on the front lines of leveraging the technology and proving value for the entire enterprise.
To help you in that effort, this white paper highlights approaches marketers can take to ensure their Generative AI investments produce measurable gains.
The paper will provide information on:
Introduction
Generative AI is one of the latest technological innovations to capture outsized attention from investors and enterprise leaders. McKinsey finds that 40% of enterprise leaders are already incorporating Generative AI into their workflows at least sometimes. Such rapid adoption has Goldman Sachs estimating that Generative AI will drive a 7% increase in global GDP and boost global productivity by 1.5% over the next ten years. Grand View Research estimates a greater than 34% compound annual growth rate for Generative AI between 2022 to 2030.
Certain functions and industries are embracing Generative AI faster than others. One of the first is marketing. According to recent research from BCG, 70% of global CMOs are already adopting it. The potential benefits of Generative AI for the marketing function seem obvious given marketing’s reliance on content to fuel conversions.
Sales and marketing executives already report optimism about using Generative AI to increase the volume and reach of paid advertising, PR, content marketing, social media, and lead generation without increasing headcount or agency spend, or adding complexity to the technology stack.
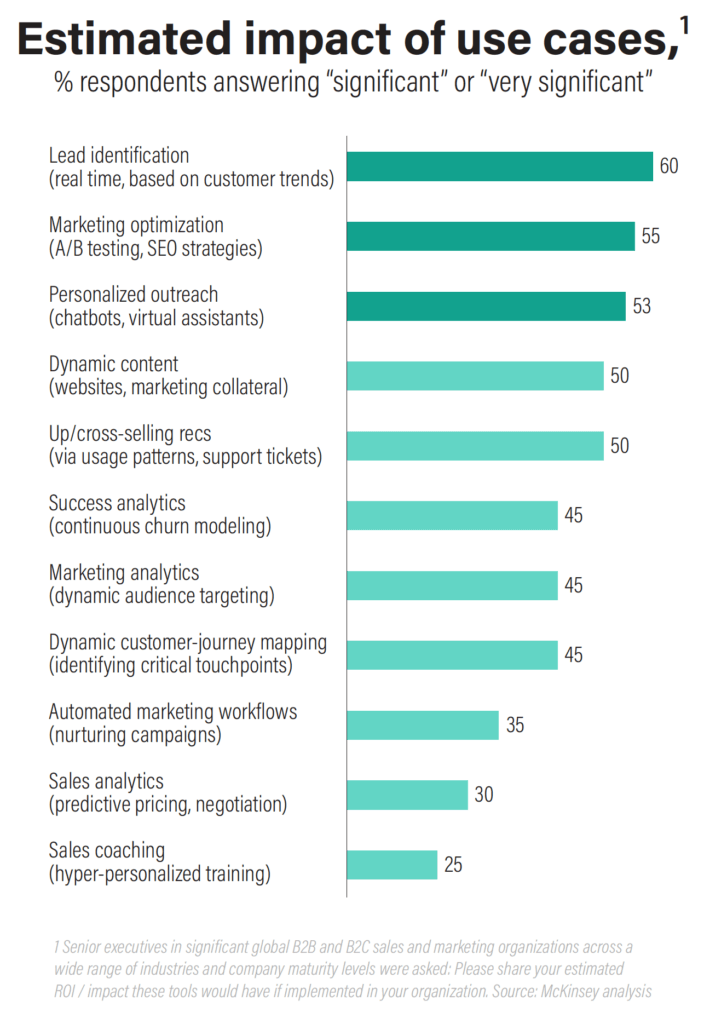
In fact, Gartner expects that by 2025, 30% of outbound marketing messages will be synthetically generated, up from less than 2% in 2022.
Yet the impact of AI-generated marketing messages on marketing results remains uncertain. The reasons why include and general lack of trust in the technology, particularly as it relates to the lack of transparency in the most popular Generative AI models, quality control issues caused by AI “hallucinations,” ongoing questions about the copyright of AI-generated content, concerns about consumer privacy and AI ethics, fear of integrating an unproven technology into the enterprise, and so on.
Furthermore, one of the reasons why Generative AI has gained such attention is its accessibility—any copywriter can sign up for ChatGPT, a well-known content-generation chatbot, and any graphic designer for DALL-E 2, a popular image generator. But if hundreds and even thousands of marketing creatives use these technologies—or others like them—in the same ways to fulfill the same tasks, the competitive advantage of the technology may be null. Put another way, while more content seems like a good thing given digital channel proliferation, flooding customer inboxes is like running in place. Marketers may keep up with the trends without increasing growth.
How can CMOs achieve impact with Generative AI? This white paper seeks to answer that question for CMOs by exploring the potential of Generative AI in marketing. We aim to equip CMOs with the information they need to steer their organizations toward uses of Generative AI that produce marketing results from increased brand equity, higher conversions and improved returns on ad spend (ROAS), to higher return on investment (ROI).
We start by exploring the evolution of Generative AI and large language models (LLMs) such as Generative Pre trained Transformer 3 (GPT-3) before discussing the current capabilities of Generative AI in marketing and the business challenges it can help marketers solve. We follow with an exploration of the point solutions that exist today for marketers and how they differentiate. Finally, we provide recommendations for how CMOs can leverage Generative AI to increase marketing efficiency and effectiveness, and deliver personalized customer experiences to achieve real results.
What is Generative AI?
How can Marketers Use It?
At its most basic level, Generative AI is a type of artificial intelligence that produces new outputs in response to instructions delivered in clear language. Though natural language processing—the category of AI under which Generative AI falls—has been around since the 1950s, recent breakthroughs in the technological models used to train AI language algorithms have made the current generation of Large Language Models, or LLMs, possible. With the advent of LLMs, Generative AI has become more accessible and usable for a larger number of users.
OpenAI’s GPT models (e.g. GPT-3 and GPT-4) are the most famous LLMs to date. Because their creator made them openly available for third-party developers to license, they serve as the underlying models for scores of solutions. The GPT models are not the only LLMs. Others include Amazon’s AlexaTM 20B, Google’s PaLM 2 (on which the more familiar Bard is based), supplements to IBM Watson, and Nvidia’s industry specific LLMs, as well as Llama 2 by Meta—these are just some examples.
The power of Generative AI lies in the amazing capacity of the underlying LLM to quickly recognize patterns in their training data and then create new content that reflects the instructions given by a (usually) human user. Put simply, LLMs are expert translators and predictors. They produce reasonable responses to prompts by translating what the prompt is asking into an associated output of the most common words or visuals.
Yet it is also important to understand what LLMs cannot do. Specifically, they cannot, as of today, understand an instruction the way humans would understand it. Nor can LLMs understand the source content on which it is trained. Instead, the LLM sees patterns in how words are often used and in what combination. This reliance on pure prediction without true understanding creates a few limitations for LLMs.
One is that they tend to “hallucinate” or make up facts. They can also generate authoritative-sounding text using information that has been disproven. Meta saw the consequences of the latter in late 2022, when it released an early version of Galactica, a LLM trained on content from medical journals. Scientific understanding is ever-evolving, but Galactica could not tell the difference between current evidence and debunked or outdated findings. As a result, the LLM mixed both when responding to prompts, resulting in texts rife with misinformation. The model was widely criticized by the scientific community and quickly shut down.
There are some simple ways for marketers to mitigate the potential for misinformation in brand content. These include writing very narrow prompts or contextualizing the prompt in a way that clearly defines the request and limits how the AI responds. Another is to implement strong fact-checking and editing standards to ensure AI-generated text and images go through a thorough robust governance review. A third is to use a Generative AI specially trained for narrow, enterprise-level use cases—these language models are often built on smaller and more specialized data sets and have human oversight which prevents off-brand language generation.
The power of Generative AI lies in the amazing capacity of the underlying LLM to quickly recognize patterns in their training data and then create new content that reflects the instructions given by a (usually) human user.
With those caveats in mind, the most common tasks marketers are using Generative AI to execute involve writing something or producing an image or video (see box below). Marketers are already using Generative AI for these purposes. Innovative marketers have also identified a range of more advanced applications. For example:
- Creating content in a way that looks beyond simply text or image generation to include SEO keyword optimized web copy, blog posts, short-form video, and other outputs. For better results, marketers can input samples of a brand’s marketing copy in order to “teach” the AI to generate language in the same brand voice.
- Optimizing creative copy to ensure high performance with a target audience
- Creating first-draft customer personas based on specific details you provide about the person’s role, industry, and business objectives
- Using synthetic customer data to train machine learning without running afoul of customer data privacy laws
- Converting brand photographs into 3D customer experiences delivered in-store or through augmented reality. Including “style transfer” prompts can teach the AI to transfer the style of one brand image or video to another to maintain the same color scheme or aesthetic.
- Identifying customer behavioral patterns and highlighting customer preferences to deliver personalized user experiences.
Looking beyond the Generative AI hype
As marketers explore ways to leverage Generative AI in their businesses, they will need to separate the hype from reality when it comes to short-term opportunities.
As of the 2022 Gartner Hype Cycle for Artificial Intelligence, Generative AI was reaching the top of the Peak of Inflated Expectations—or Peak Hype, if you will.
Other technologies have received similar early attention. For example, think of recent excitement about the Metaverse or Blockchain. Both technologies were widely heralded for their transformative potential, but have failed so far to deliver on it. This pattern is so common that the advisory company Gartner coined a name for it back in the mid-1980s—the “hype cycle.”
Hype cycles consist of five phases. The most important for the context of Generative AI include the second phase, or the Peak of Inflated Expectations—when a technology attracts massive funding, startups proliferate, and experts tout its promise—and the third, the Trough of Disillusionment—when early experiments fail to live up to their promise and the market begins to question its utility (the Metaverse and Blockchain are currently in the Trough).

Gartner predicts that Generative AI will reach the point of mainstream adoption and value return within two to five years. That fast velocity should encourage CMOs to invest now and explore ways to drive benefits in their organization. But be aware that between Peak Hype and full productivity, there is the potential for missteps and unfulfilled expectations.
Considering the range of possible use cases for Generative AI in marketing, it would be very easy to invest widely and without a clear idea of what success looks like. To optimize the positive outcomes and minimize the negative, CMOs should instead concentrate Generative AI investments on marketing’s biggest challenges or opportunities. First among them? Driving growth.
How Generative AI Can Increase Marketing’s Impact
Delivering growth will require marketing teams to produce stronger campaign and branding returns with the same level and distribution of investment.
CEOs are looking to their heads of marketing to play a prominent role in delivering growth. CMOs are responding to that call to action with a back to basics marketing strategy that places campaign creation and management, brand strategy, and effective marketing operations at the top of the priority list.
Marketing budgets have barely returned to their pre-pandemic level of about 10% of revenue—allocated on average more-or-less evenly across paid media, in-house talent, agencies and services, and technology (see Figure). Delivering growth will require marketing teams to produce stronger campaign and branding returns with the same level and distribution of investment. Yet when you ask them, many CMOs feel that their ability to overperform in today’s market is partly constrained by a lack of talent.
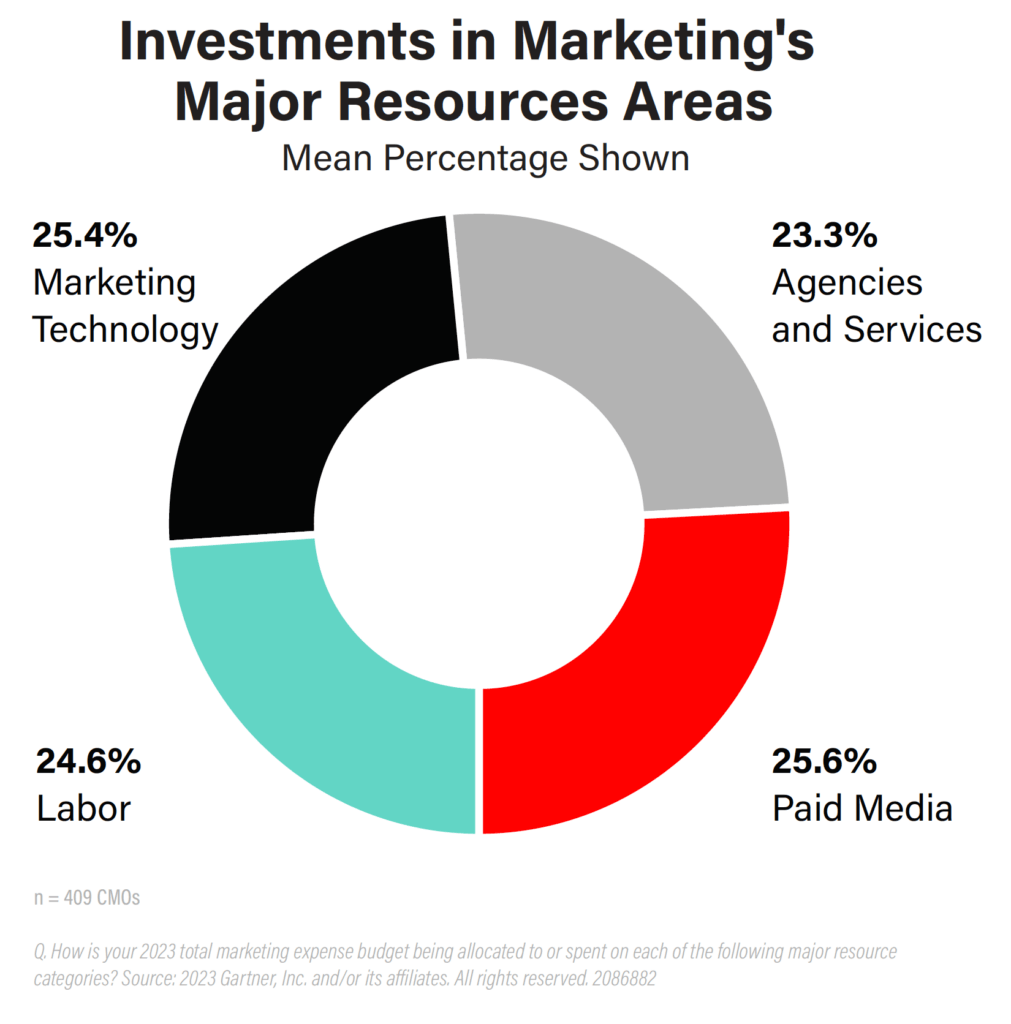
Generative AI’s key capabilities related to language- and image-generation are well-aligned with these priorities of campaign creation and brand strategy and to the need to make the most of limited talent resources. Generative AI solutions that can help marketers create either more content or better content will be key assets in moving the needle on marketing’s most important key performance indicators (KPIs). These include classic metrics such as higher return on ad spend (ROAS), lower customer acquisition costs (CAC), greater conversion rate optimization, higher purchase completion, lower cart abandonment, and higher lifetime value of a customer (LTV).
More sophisticated marketing organizations may use multi-factor metrics—the market research and consulting firm Kantar advocates for metrics like pricing power (can you demand a price premium) or activation power (how well you convert a customer’s predisposition into a purchase).
Regardless of your preferred metrics, marketers have two primary and complementary ways to improve them: greater efficiency and greater effectiveness.
Improving efficiency and effectiveness with Generative AI
Business leaders often ask two central but distinct questions when evaluating new technology investments. CMOs exploring Generative AI are no different. The questions are:
- Will this investment help me do more of what I already do and/or get to do work that typically doesn’t get done, using the same budget size and allocation?
- Will this investment help me improve the results from the work I do?
The Benefits of Greater Efficiency Using Generative AI
The first question about doing more with the resources you have—including budget and talent—is primarily a question of efficiency. That word “efficient” can generate mixed reactions in business circles. Ambitious CMOs may view pursuing efficiency as less exciting than pursuing innovation, for one. For another, suggesting that a team is inefficient implies blame, as if the team members are knowingly doing their work in a suboptimal way.
Increased efficiency opens up marketing capacity to do more work, which can also enable more innovative work.
We have a different take on efficiency as a key enabler of profitability and innovation. Increased efficiency opens up marketing capacity to do more work, which can also enable more innovative work. Since every CMO has had to focus on a tight set of marketing priorities that fit within their limited budgets and resources, some initiatives will fall outside those priorities because of a lack of capacity or budget to do it. When you improve efficiency, you increase capacity, and create the potential to expand your priority list.
From this perspective, efficiency can lead to growth, whereas a lack of efficiency can severely restrain results. In fact, more than half of the respondents to the PwC 26th Annual Global CEO Survey name labor shortages as a threat to their firm’s 10-year survival—the third most common survival threat after changes in customer demand and regulation. Greater efficiency delivers a competitive advantage by enabling you to get more out of the talent you have.
In marketing, greater efficiency can translate to generating leads and retaining customers with less labor and waste, resulting in the same outputs at lower cost. Even before Generative AI became part of the conversation, marketers were actively looking for efficiency gains. The rising importance placed on marketing operations over the past few years offers one proof point. Marketers that leverage Generative AI with an eye on efficiency can automate manual tasks and reduce the burden of repetitive, error-prone tasks. Ultimately, this frees up marketers’ time to focus on other high-value revenue-generating activities and achieve faster time-to-market.
Generative AI is already being touted for its potential here. A new study by the National Bureau of Economic Research, for example, found that Generative AI boosts worker productivity by 14%. Copywriters report anecdotally that using a self-service Generative AI chatbot, like ChatGPT, or a marketing copy point solution, like Copy.ai, helps them develop ideas, outlines, or even first drafts. These platforms also speed up the process of producing derivative outputs, such as social copy to promote an eBook. In this latter example, the copywriter can “train” the AI in the style and messages of the eBook before prompting it to generate related social messages.
The Benefits of Greater Effectiveness Using Generative AI
Efficiency is not the only goal marketing leaders have for their function, as noted. In fact, the benefits of greater efficiency can erode if the additional marketing outputs fail to produce the same performance levels. Greater results is not an efficiency output but rather one of effectiveness—in other words, campaigns or branding that drive more new customer acquisition, more purchases from existing customers, and/or larger purchases. CMOs that focus on effectiveness are looking to answer that second question of improving results from the work that they do.
As with efficiency, Generative AI has potential to drive greater marketing effectiveness through different mechanisms. While efficiency can come about by helping marketing teams do more of the same activities, effectiveness results from Generative AI helping marketers get better results from the same activities. For example, if a Generative AI application has been trained to leverage consumer insights and past campaign performance data, it can optimize campaign language to outperforms human-generated variations. The result is more revenue than from campaigns that were solely human-generated.
Marketers are not faced with a binary choice between efficiency and effectiveness. They want both. Yet in a world of choices and limited priorities, prioritizing effectiveness from Generative AI is likely to produce greater ROI.
To understand why, consider the data on what makes a good return on investment from digital marketing. Quantified by Adobe, a good return is calculated as a $5 return for every $1 spent over six to twelve months. Using that math, $1 million invested in digital marketing campaigns is considered a good investment if it produces $5 million in benefits or returns.
Generative AI has potential to drive greater marketing effectiveness by helping marketers get better results from the same activities.
Yet maximizing efficiency on a campaign with a $1 million budget produces by definition only savings on the original $1 million investment. As a result, efficiency gains top out as the maximum of the invested spend (and likely lower, since you have to spend something to get efficiencies out of it). In other words, you can get more efficient with your $1 million investment, but the benefits still max out at $1 million.
By contrast, there is no upper limit to the possible return on a more effective digital campaign. Assuming a 5-to-1 “good” return, a more effective digital marketing campaign could return $5 million off the $1 million investment, 5X the return from being more efficient.
Marrying Efficiency and Effectiveness to Enable Personalization
Marketers don’t have to make an either-or choice between efficiency and effectiveness. In fact, when marketers unlock improvements in both efficiency and effectiveness they make possible one of the key long-term goals of marketing—personalization.
Consumer research consistently finds that consumers increasingly expect personalization and exhibit more loyalty to brands that provide it. Over half of customers also say that they will become repeat buyers after a personalized experience. That’s one reason why personalization initiatives saw a 7% increase year-over-year in 2022, according to a report by Twilio Segment.
Generative AI could produce multiple versions of the same video on the same subject, each one optimized to appeal to a specific customer group. That’s the direction of Generative AI for personalized marketing.
Of course, personalization is not new. Marketers have viewed it as a strategic imperative for at least a decade. Yet they have also faced significant barriers in delivering it. Those barriers include challenges extracting insights from customer data and analytics and a lack of creative talent to produce content at scale. Even brands that have matured their customer data analytics programs have had limited ability to activate that data due to limits in their creative capacity.
Generative AI has the potential to change that. Solutions like Persado already exist and are market-tested to understand customer segment data and generate distinct messages for different customer groups. Using personalized messages, brands are able to tailor customer experiences in a way that enables brands to build stronger customer relationships and drive higher levels of engagement and conversions.
Consider how this relationship between efficiency, effectiveness, and personalization could operate for the creation of a marketing asset like a video. A general-purpose, core LLM could help marketing teams develop video assets more efficiently—meaning in less time, therefore using less human labor. Video avatars and voice overs are being adopted rapidly and feel much more human than they did in the past. Using them instead of human actors for certain assets results in a highly efficient output, but it is not necessarily more effective. Nor is it more personal, since it is still one video designed to appeal to as many people as possible.
If that video-focused Generative AI solution were trained to speak in the brand voice of the company, however, the brand would maintain the efficiency benefits and increase effectiveness (assuming, of course, that the brand voice is more engaging for loyal customers). Take it one step further and train the Generative AI with data on what makes a video more engaging and high-performing, and the technology could generate a single video that produces more conversions. Train it further with customer segment data, and the Generative AI could produce multiple versions of the same video on the same subject, each one optimized to appeal to a specific customer group. That’s the direction of Generative AI for personalized marketing.
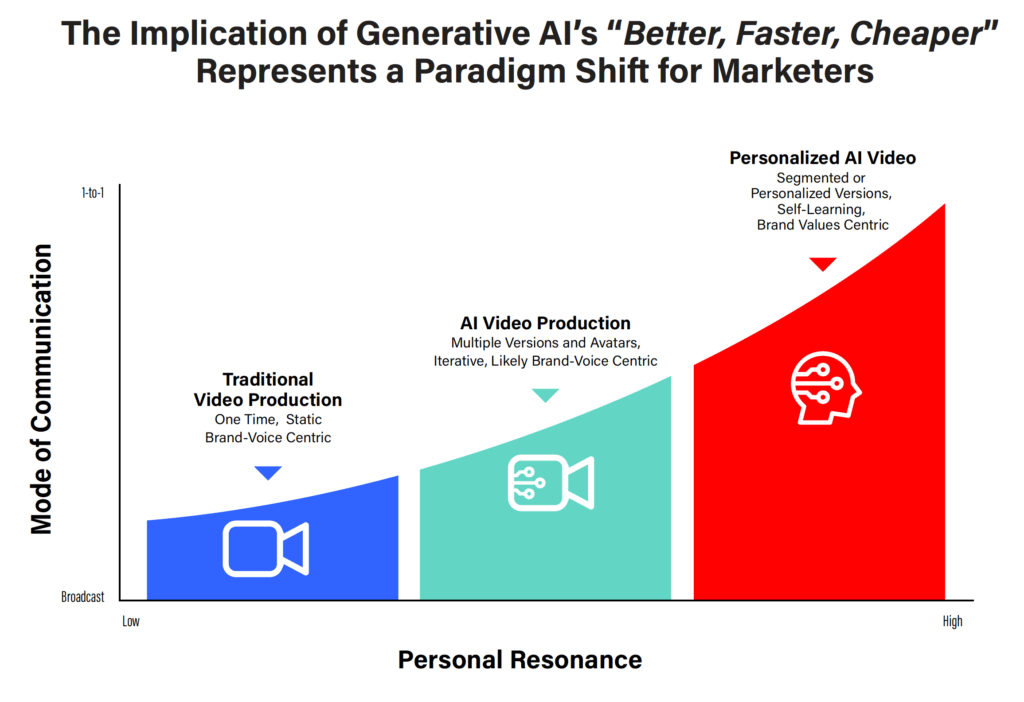
Personalization represents a new frontier of possibility for marketing-led growth, and AI is at the forefront of enabling it. Twilio Segment, for example, reports that more than 9 in 10 companies (92%) are using AI-driven personalization to drive business growth. Generative AI will be a key part of that personalization technology stack.
The key for marketers that want to benefit through efficiency and effectiveness, and thereby set the stage for personalization, is to choose a Generative AI solution with the necessary capabilities to meet their marketing goals.
Choosing Generative AI That Fits the Marketing Goal
Since mid-2021, when OpenAI made its GPT-3 LLM commercially available (the company has since launched GPT-4), scores of Generative AI solutions have come onto the market.
OpenAI itself offers focused solutions for language generation, image generation and code generation. They are ChatGPT, DALL-E, and Codex, respectively. OpenAI also sells licenses to end-user organizations using it to build proprietary point solutions and to startups that sell point solutions with features that add value on top of the core GPT capabilities. Some of the better-known examples in marketing are Copy.ai, Jasper.ai, Writer, and others. Nvidia has also licensed its LLM for third-party developer adoption as has Amazon for its customer base.
Beyond OpenAI-enabled solutions, many large technology companies have integrated proprietary Generative AI capabilities into their platforms. Examples include Adobe Firefly, which enhances visual editing within the Adobe Creative Suite.
In short, the universe of Generative AI options available for marketing teams is vast and growing. Chances are, you already have—or will soon have—some Generative AI capabilities available in your existing martech stack.
CMOs will need to set guidelines for Generative AI adoption to ensure the organization has the right solution to meet its goals.
In addition to the enterprise-focused solutions becoming available, there are also many consumer-focused, self-service solutions like ChatGPT, DALL-E, Midjourney, and others that marketing team members may be using to help with their work, even without your businesses officially licensing it. The challenge for CMOs will be to set guidelines for Generative AI adoption and ensure you have the right solution to meet your goals.
As Generative AI matures and businesses get more experience using it, the market is likely to shift away from the dominance of core LLMs and diversify with a range of point solutions trained to address specific marketing use cases.
Writer.com, for example, is an enterprise AI platform that helps businesses create marketing content including content marketing, demand generation, product marketing, brand marketing, and content design. Marketers can use it to generate, improve, and edit content for various industries such as financial services, healthcare & life sciences, e-commerce & retail, and technology. The AI solution allows businesses to save time, streamline workflows, and generate content.
More specialized Generative AI point solutions will emerge for specific use cases in marketing. Table 1 includes a non-exhaustive list of available solutions that promise certain advantages for marketers over core Generative AI solutions.
For example, one of the most widely used Generative AI solutions to date is ChatGPT, which had more than a million registered users within a month of becoming commercially available in November 2022. ChatGPT has demonstrated its ability to generate relevant, intelligent responses to prompts, and even improve subsequent drafts based on clear guidance about tone and details. It can improve efficiency.
ChatGPT has not been trained to influence consumer behavior or drive conversions, however. In other words, while ChatGPT helps marketers with the challenge of efficiency, it does little to improve effectiveness. If a marketer wants to leverage Generative AI to drive more effective campaign performance, therefore, it would need a Generative AI solution trained to do so. As far as we know, Persado Motivation AI—a specialized, enterprise Generative AI solution trained on over 1.5 billion customer campaign interactions from 150 million US customers—is the only one purpose-fit to drive digital marketing campaign performance.
To reiterate, the choice between efficiency and effectiveness is not binary. Some Generative AI marketing investments will be focused on efficiency and some on effectiveness and some will optimize both—enabling that next-wave push toward personalization. Achieving a balance will require CMOs to choose Generative AI solutions trained for the specific goal they are trying to achieve.
Consider a typical interaction between a buyer and a sales agent. If marketers want to generate intelligible scripts that agents can use to quickly respond to inquiries from prospects, they may use a core Generative AI solution like ChatGPT. These scripts can help the agents be more efficient and ensure that they represent a product or service consistently and accurately.
If marketing wants to craft scripts that optimize language in a way that motivates a caller to take a specific action, however, it needs a Generative AI specially trained to tap into customer motivation so that those sales interactions result in higher conversions.
Generative AI point solutions for marketing use cases
As Generative AI matures and businesses get more experience using it, the market is likely to shift away from the dominance of core LLMs and diversify with a range of point solutions trained to address specific marketing use cases.
Writer.com, for example, is an enterprise AI platform that helps businesses create marketing content including content marketing, demand generation, product marketing, brand marketing, and content design. Marketers can use it to generate, improve, and edit content for various industries such as financial services, healthcare & life sciences, e-commerce & retail, and technology. The AI solution allows businesses to save time, streamline workflows, and generate content.
More specialized Generative AI point solutions will emerge for specific use cases in marketing. Table 1 includes a non-exhaustive list of available solutions that promise certain advantages for marketers over core Generative AI solutions.
Table 1: Generative AI Marketing Solution Providers | |
Vendor | Use Cases |
Anyword | Ad copy, blog post, email copy, social post, meta description, product description, AI writer tool, and more |
Copy.ai | SEO content creation, ad copywriting, conversion rate optimization, social listening, review response writing, customer support, translation and language localization, dynamic pricing, churn prediction and prevention, and email copywriting |
D-ID | Video (live and speak portrait), and avatars |
Descript | Video editing, podcasting, screen recording, transcription, clip creation, and AI voices |
Jasper.ai | Content, product descriptions, email marketing, webinar, blog post, and social media content |
Lyne.ai | Cold email acquisition, website design, outsourcing, and SEO |
Memorable.IO | Optimization, image generation, creative strategy, and ad make |
Mutiny | Website conversion, account-based marketing, scale paid acquisition, product-led growth, and website A/B testing |
Optimizely | Email campaigns, personalization, content marketing, digital asset management, and more |
Pencil | AI-generated ad creative, ad copy, static and video creative, and more |
Peppertype.ai | Content writing, video production, and language translation |
Persado | Cart abandonment, acquisition, onboarding, retention, pre-collections, and customer service |
Recast | Image generation |
Talkoot | AI copywriting, project management, campaigns, multi-language localization, and more |
Writer | Marketing, content strategy, editorial, support, documentation, learning and development, operations and IT, and human resources |
Four areas where Generative AI point solutions must optimize
For point solutions to differentiate themselves and justify licensing costs, they will need to provide improvements and value-add above the capabilities that enterprises can get from core LLMs. In the short term these improvements will fall into a number of key categories:
- Optimized user interface and user experience. Effective point solutions deliver a seamless and straightforward experience for end users, so they can easily use it and deliver value. Prompting, for example, should be streamlined, automated, or delivered with white-glove service.
- Workflow integration. Point solutions integrate into your enterprise processes and martech stack in a way that allows marketers to integrate into existing workflows.
- Metrics-based reporting. Point solutions have built-in reporting and data generation that provide insights on target metrics, so that marketers can leverage the insights or quantify value.
- Extension of the core tech. Point solutions need a defensible extension and value-add beyond the core technologies so that it can serve specific needs and use cases. Those extensions also create a distinct value proposition that would be hard for a competitor to replicate in the same way.
Starting recommendations for Generative AI in marketing
Given the current market enthusiasm, the state of Generative AI at peak hype, and the considerations around efficiency and effectiveness, where should CMOs explore the technology and focus their investments? We recommend the following actions:
Examine marketing’s current strategic goals and the barriers to achieving them. By focusing on marketing’s top goals, you are likely to deliver bigger marketing outcomes while staying within the same marketing budget.
Given the combination of Generative AI’s present day use cases around language, images, and video, and the large share of spending that goes to talent and to paid advertising, marketers will likely find their biggest opportunities in labor efficiencies and greater returns from paid advertising. Identify concrete areas within which to experiment. These could be content generation, workflow task automation, customer segmentation, or marketing personalization, to name a few.
Take an experimental eye to early uses of Generative AI and set up conditions to allow you to measure impact. Take different approaches to efforts to become more efficient, more effective, or both. Tie these initiatives to concrete metrics such as ROAS (Return on Ad Spend), CAC (Customer Acquisition Cost), and LTV (Lifetime Value of a Customer).
Seek opportunities to deliver personalization at scale using Generative AI to provide customized content to the right customer at the right time as a way to achieve higher conversions.
Identify point solution providers that align with specific business needs and have a proven track record of working in enterprises to deliver higher ROI.
The future of personalization and Generative AI in the next 24 to 36 months looks promising. The marketing function represents one of the first areas of the business that will actively adopt the technology and deliver measurable results through personalized marketing campaigns, improved customer engagement, and concrete revenue growth. As the category continues to evolve, we can expect to see more sophisticated solutions and use cases that generate revenue across various industries.